The MVP for a model serving cloud-native solution.
At my previous role, we actively set up a production environment for deploying hundreds of machine learning models. I want to talk about the central metric in this task - RPS (requests per second) - and the first step taken to improve it.
Typically, RPS is associated with Site Reliability Engineering. Often, it involves web services with heavy backends, sprawling databases, and a large pool of users. For example, the SRE team at Google recently exceeded 3,500 people. Ben Treynor, VP of SRE at Google, explains: “Typically, we hire about a 50-50 mix of people who have more of a software background and people who have more of a systems engineering background. It seems to be a really good mix.”
In the field of machine learning, I would highlight some preliminary knowledge required for developing such an ML environment:
- DevOps and infrastructure development;
- Building end-to-end ML pipelines: from data preprocessing and feature selection to parameter tuning;
- Understanding the open-source world of model deployment and how to leverage it to avoid reinventing the wheel.
Ultimately, it becomes a mix of hardcore engineering, non-trivial analytics, and system design. It sounds inspiring until you start measuring the system’s performance.
🐌 RPS = 21
In its most basic version, we achieved 21 requests per second, or a minute-long wait for a response under a load of around 1200 users. Unacceptable. We need to identify the bottleneck.
In the image below, the folks at MLflow propose a model storage architecture. AWS S3 is used for storing the weights. However, data transfer occurs over the network (a.k.a. slowly).
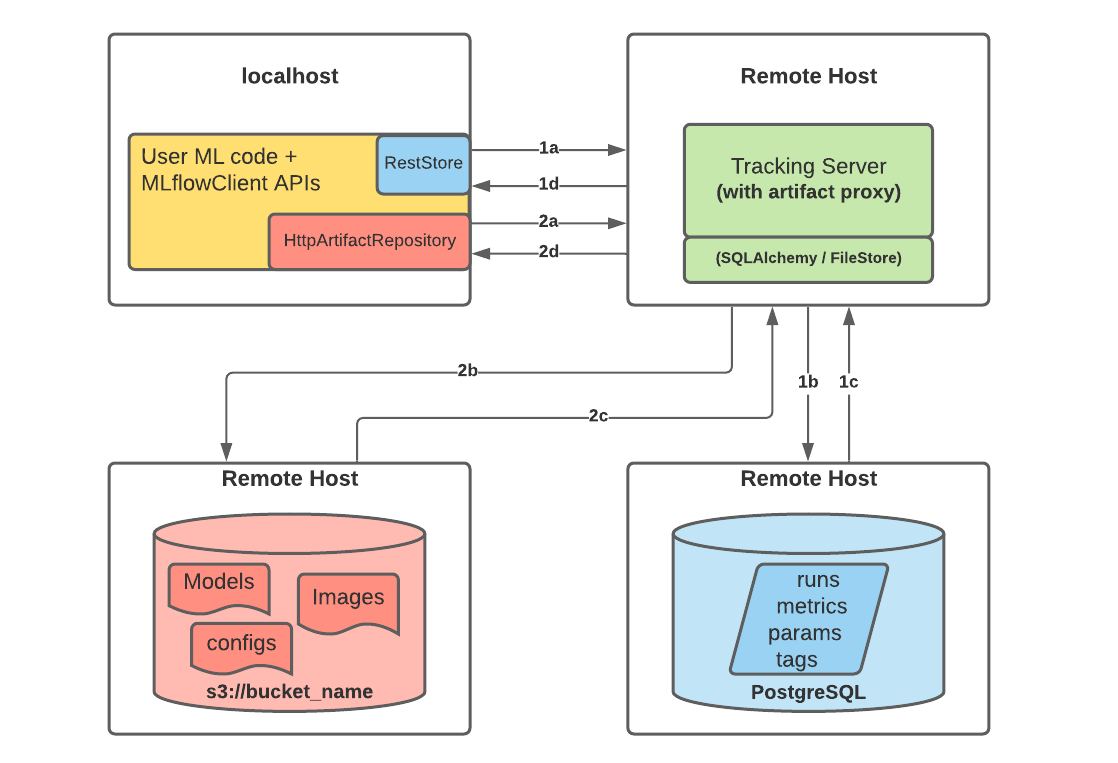
It was noticed that the model weights were being transmitted over the network with every request to the environment, which was a severe bottleneck. Let’s keep the models in RAM after the initial transfer.
🏃♂️ RPS = 155
A simple heuristic, yet the acceleration is more than sevenfold. In the end, through a trade-off between memory and speed, we achieved acceptable system performance for the first version of the product. This means we can proudly call ourselves MLOps engineers.